In the 2023 State of AI in Engineering study conducted by Forrester Consulting, more than two thirds of US and European automotive engineering leaders stated they need to find new ways to speed up the ideation and launch of complex new products like battery electric vehicles (BEVs) in order to stay competitive.
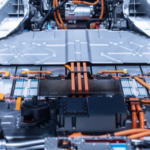
In the discussion surrounding the battery value chain, the spotlight frequently falls upon the sources and provisions of battery materials and components. Nevertheless, an emerging challenge within the automotive sector is notable: the ability to assess batteries to guarantee exceptional performance, durability, and safety.
In a landscape marked by surging consumer demands and competitive pressures to enhance range and charging efficiency, these teams are grappling with a host of challenges as they navigate the path to product launches.
Paramount among these challenges are concerns regarding battery longevity, protracted charging periods, and limited operational range. These aspects present not only hurdles but also opportunities where machine learning and AI can make a significant impact.
Battery testing is extremely complex with thousands of design scenarios that all require a very long time to test.
The testing dilemma
As the physics of complex products like batteries become more and more intricate and time-consuming to understand, engineers find themselves in a dilemma; they either conduct excessive tests to cover all possible operating conditions, or run insufficient tests that risk the omission of critical performance parameters.
One is time-intensive and risks staying competitive, while the other risks brand reputation and customer safety.
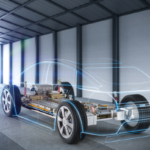
Through conversations with battery R&D leaders at many global OEMs, it has become evident that engineers are feeling increased pressure when it comes to guaranteeing the thermal performance – as well as the life and health – of BEVs in real-world scenarios.
Every OEM has the challenge of specifying and developing the best battery system for their technology, and they need to go to market with total confidence in how it performs. We know that engineering a battery pack requires a huge amount of testing – years in some cases – because it’s a complex new technology and in many areas unproven in the field.
Balancing key characteristics of an EV battery system such as range, re-charge rate, and lifetime requires months of validation testing across thousands of scenarios and conditions.
Machine learning
Many of Monolith AI’s clients perform battery tests across 1000s of channels, generating terabytes of data per week. They’re running out of test stands and don’t know what optimal tests to run, and don’t have the ability to learn from this vast amount of data as quickly as they need.
This is where Machine Learning (ML) comes in.
Through the ability to learn from data, test engineers can understand behaviour characteristics that are so complex, that without the right tools it is incredibly difficult to decipher. ML that learns from real world test data is a reliable and effective means for solving the intractable physics of batteries that current simulation and test planning tools don’t efficiently solve.
Furthermore, employing machine learning for projecting battery lifespan provides greater precision in contrast to conventional approaches like equivalent circuit models (ECMs).
Standard models such as ECMs tend to show higher degrees of inaccuracy compared to the generally lower inaccuracies observed in ML models.
ECMs are currently still used because they’re easier to embed into operating systems, but it’s well-known that they have a higher degree of inaccuracy. Most battery labs are trying to find ways of incorporating ML tools into their durability models because it’s more accurate and results in better-performing batteries.
Less is more
Monolith AI recently released a major product update based on a new ML approach called Next Test Recommender (NTR), built on a robust active learning algorithm.
NTR gives active recommendations on the validation tests to run during the development of hard-to-model, nonlinear products such as batteries and fuel cells, and found that AI can reduce testing by up to 73%.
In one fuel cell use case, an engineer trying to configure a fan to provide optimal cooling for all driving conditions had a test plan for this highly complex application that included running a series of 129 tests. When this test plan was inserted into our software, it returned a ranked list of what tests should be carried out first.
Out of 129 tests, the platform recommended the last test – number 129 – should actually be among the first five to run and that 60 tests were sufficient to characterise the full performance of the fan, representing a 53% reduction in testing.
The promise of AI, therefore, is simple: test plan optimisation that offers greater R&D efficiency and faster time-to-market.
For the electric car industry, this means speeding the development and integration of batteries, and for customers a faster and safer transition to electric vehicles.